Why is Data Driven Change Management Important?
If data science was a tool in a construction tool belt, it would be the measuring tape. During the change management (CM) process, data science measures employees’ behavioral changes, change readiness markers, goal achievement, implementation timelines, leadership tactic effectiveness, among other potential change indicators. As this reference set grows, so does the ease of predictive organizational change models (HBR, 2017) which help companies understand which actions will accelerate adoption of new behaviors, practices, and processes.
However, despite data’s undisputed (Deloitte, 2022) and expanding CM utility, data is often underused in the CM process. A leader forging change without data is like a home contractor building without a measuring tape. In our recent Expert Insight Series interview, industrial psychologist Dain Johnson reflected, “Despite the tools available, people still use their intuition to make people decisions. There’s a better way.”
What is the better way to lead change?
Recent research by Deloitte suggests that incorporating data into the CM process drives better change outcomes. In fact, “We know from our research that high performing organizations are 3.5 times more likely to use data to inform change efforts” (Deloitte, 2022).
Deloitte’s latest research drives change with a four-step data model, “Transformation Intelligence™”. Rather than relying on human intuition, data is incorporated into every stage of the change process.
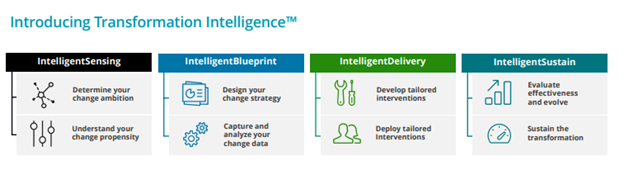
(Source: Deloitte, 2022)
What’s the best place to start using data in change management?
Many organizations may not be ready for full Transformation Intelligence™, but they can start by incorporating practical tools to support change adoption. For example, automated dashboards tracking key metrics can help drive an objective change process through transparency and data visibility (HBR, 2017) and app-based training tools can improve employee adoption of new processes (Deloitte, 2022).
Equipped with the right tools, leaders can make decisions based on users’ behavior rather than relying solely on intuition. Business intelligence dashboards allow companies to track quantitative employee behaviors (i.e., adoption levels of newly deployed software) in addition to qualitative survey data, and technologies such as data analytics, AI, and ML take it a step further by offering predictive modeling to forecast the effectiveness of leadership decisions within different subsets of people, including geographies and cultures (Deloitte, 2022).
An intentional integration of data into change management efforts will position businesses to be more agile in navigating inevitable changes ahead.