What is a Data Lake?
A Data Lake is a centralized repository for all manner of structured, semi-structured, and unstructured data. It stores data in its original format with no transforming or cleansing and can cost-effectively scale to meet enterprise organizations’ needs.
A Data Lake’s primary goal is to provide data scientists and analysts a single repository of all the organization’s data for deep analysis. Data Lakes are rising in popularity, with some market analysts giving it nearly 33% of the value chain market.
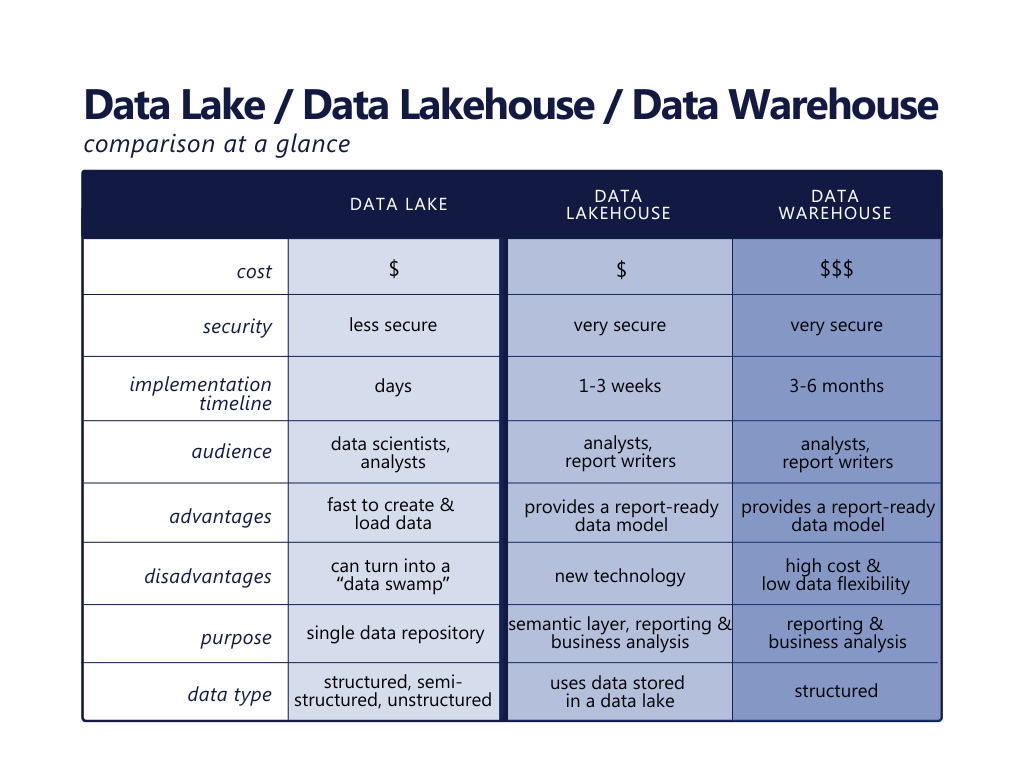
What is a Data Lakehouse?
A data lakehouse brings order to a data lake by enabling a modeled, relational representation of data stored in a data lake. It combines the flexibility and scalability of a data lake with the structure and management features of a data warehouse all in a simple, open platform. In contrast to a traditional data warehouse, a data lakehouse utilizes data from a data lake without requiring a copy of the data, thus reducing data redundancy.
A data lakehouse’s primary goal is to provide analysts and report writers with a semantic, reportable layer of data. They can use this data to combine disparate data sets and build and distribute reports to support organizational operations.
Benefits of a Data Lake + Data Lakehouse
Implementing a data lake enables an organization to create a centralized repository of their data quickly. Loading data to the data lake is straightforward, only requiring a connection to the source data. Instead of the traditional ETL (Extract, Transform, Load) framework, a data lakehouse is implemented using ELT (Extract, Load, Transform). Transformation of the data is completed by analysts, scientists, and report writers after the data lake is established. A data lake makes data available quickly, with the caveat that analysts and data scientists will later need to do the hard work of scrubbing and modeling the data for effective reporting and analysis.
Cloud storage is inexpensive, making enterprise-use of cloud storage infrastructure a growing trend2. Specifically, a data lake and lakehouse in the cloud can be a more cost-effective option than traditional data warehouse storage. For example, Microsoft Azure offers five terabytes (TB) of data storage for $200 per month3. Comparatively, five TB of data storage in a Data Warehouse (e.g., in Azure’s Synapse Analytics) could cost as much as $1,200/mo4.
Data lakes offer support for Machine learning (ML) and Artificial Intelligence (AI). Azure provides tools that make ML and AI quick and easy to implement. Big data processing tools such as Hadoop and Spark can also be deployed on top of data lake, making it a valuable asset for predictive and diagnostic analytics.
Data scientists, analysts, and report writers can connect to either raw or modeled, relational data with various analytical tools including Power BI to visualize data. With Azure Data Lake and Lakehouse, users can take advantage of native Power BI connectors to quickly find the data files they need for their analysis.
The design of your data lake will be driven by the data available, rather than the specific reporting requirements – which can be cumbersome to define – or the available technology, which may change with time. Importing new data to a data lake is simply a matter of moving data, making it an expedient way to provide data access to data scientists and analysts.
In Summary…
The primary benefits of a data lake are centralized data, and wide reporting support. Though these benefits have been shown to enable an increase in organizational growth5, the data lake alone should not be considered a replacement for a traditional data warehouse. However, a data lake paired with the computational power of a data lakehouse can support the operational and analytical needs of most organizations at a fraction of the cost of a traditional data warehouse.
References
- Data Lake Market to hit US $24,308 million by 2025 (2020) [Market Research]. Adroit Market Research https://www.globenewswire.com/news-release/2020/11/24/2132790/0/en/Data-Lake-Market-to-hit-US-24-308-0-million-by-2025-Global-Insights-on-Trends-Value-Chain-Analysis-Leading-Players-Growth-Divers-Key-Opportunity-and-Future-Outlook-Adroit-Market-Re.html
- Data Storage Trends in 2020 and Beyond (2019) [White Paper]. Spiceworks https://www.spiceworks.com/marketing/reports/storage-trends-in-2020-and-beyond/
- Microsoft Azure Storage Overview Pricing (2021) [Service Offering]. Microsoft https://azure.microsoft.com/en-us/pricing/details/storage/
- Microsoft Azure Synapse Analytics Pricing (2021) [Service Offering]/ Microsoft https://azure.microsoft.com/en-us/pricing/details/synapse-analytics/
- Angling For Insight in Today’s Data Lake (2017) [Analysis Report] Michael Lock, Senior Vice President, Analytics and Business Intelligence (Aberdeen) https://s3-ap-southeast-1.amazonaws.com/mktg-apac/Big+Data+Refresh+Q4+Campaign/Aberdeen+Research+-+Angling+for+Insights+in+Today’s+Data+Lake.pdf